Blog
ZIZI: QUEERING DATASETS AND LATENT SPACES – JAKE ELWES
Zizi – Queering the Database aims to tackle the lack of representation and diversity in training image datasets of people’s faces used by facial recognition systems. These datasets often have a bias towards heteronormativity and male western faces. The resulting systems frequently fail to recognise women of colour, trans people and other marginalised communities due to the (usually unconscious) biases of the people gathering the input data and training these systems.
One way to examine what a neural network has learnt is to use a ‘generative adversarial network’ (GAN). This allows you to create and visualise new images (in this case faces) which don’t exist in the original dataset but have been constructed from what the A.I. has learnt. This is also the basis of deep-fake technology, where this is taken a stage further and these ‘fake’ faces can be manipulated and controlled.
In this work, a GAN trained on a standardised open-source dataset of 70,000 images of faces has been disrupted with the addition of 1000 images of drag kings, drag queens and drag things. This shifts the resulting ‘fake’ faces away from normativity and into a space of queerness. Faces become less recognisable and realistic, with exaggerated makeup and gender-fluid features confusing and breaking down the system, posing the question: do we as a queer community want to be recognised by these systems or do we want to mess with them and confuse them?
In machine learning the limitations, boundaries and space containing everything a neural network has learnt is called a ‘latent space’. These digital spaces are reflections of social and public spaces and they are affected and limited by the data with which we feed these systems. We can shape these spaces to reflect the world we want, rather than the world we have, by carefully selecting what we feed it.
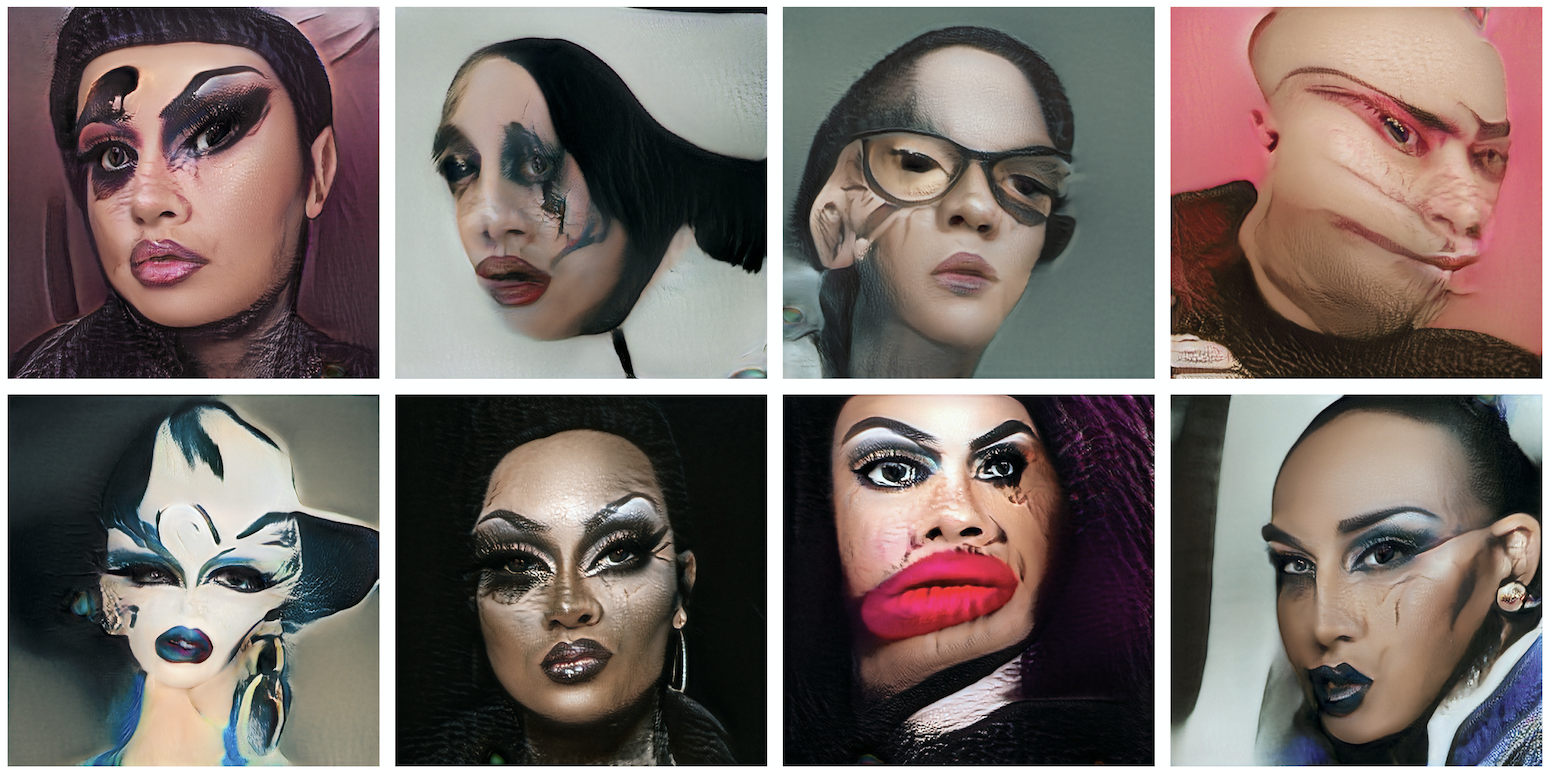
In many ways, I believe that there is already an inherent queerness to latent space. While an algorithm may have been given the task to distinguish between male and female faces – due to the labels inputted by humans – the neural network in fact reduces input faces to numbers or coordinates in a latent space. If we remove our human classifications and binaries from this system, it doesn’t read images of faces as male or female. Instead, everything falls into spectrums or points in this fluid and continuous space. Binaries and labels can be read back into these systems depending on where in this space an image is recognised to be. But we can also explore the unmediated mathematical latent space. Zizi – Queering the Dataset takes an unsupervised journey through this space. In the video, you can watch as it continuously and effortlessly moves through different identities and personas.
These ideas have extended into a wider project, exploring the relationship between drag and A.I. For example, in The Zizi Show (2020) a community of drag artists have been invited to virtually perform in each other’s bodies constructing a virtual cabaret show.
The Zizi Project is ongoing and expansive. The first stage was to use a dataset of drag identities to challenge these systems. Moving forward the project has become a collaboration with a diverse range of UK drag artists. We want to consider how deep-fake technology can be used in a new way as a performance tool. We also aim to reclaim this technology which can be oppressive and exploitative, instead using it to celebrate the queer community in a playful, consensual and accessible way.
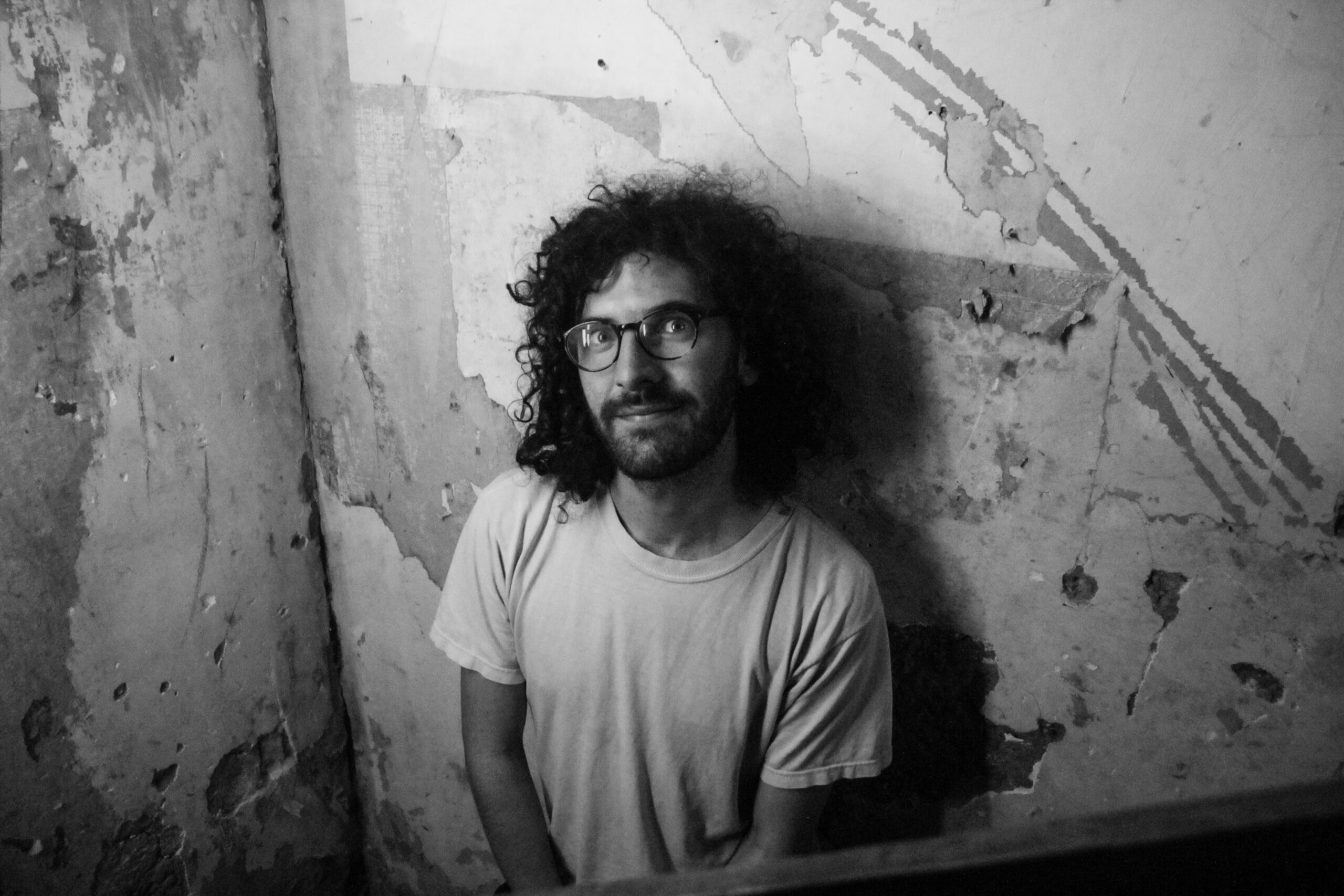
Jake Elwes is a media artist living and working in London. He studied at The Slade School of Fine Art, UCL (2013-17). Recent works explore his research into machine learning and artificial intelligence. His practice looks for poetry and narrative in the success and failures of these systems, while also investigating and questioning the code and ethics behind them.